SDF
AI Sql Development Tool
Introducing SDF: The AI SQL development tool for 10X faster development speed.
view tool
Share
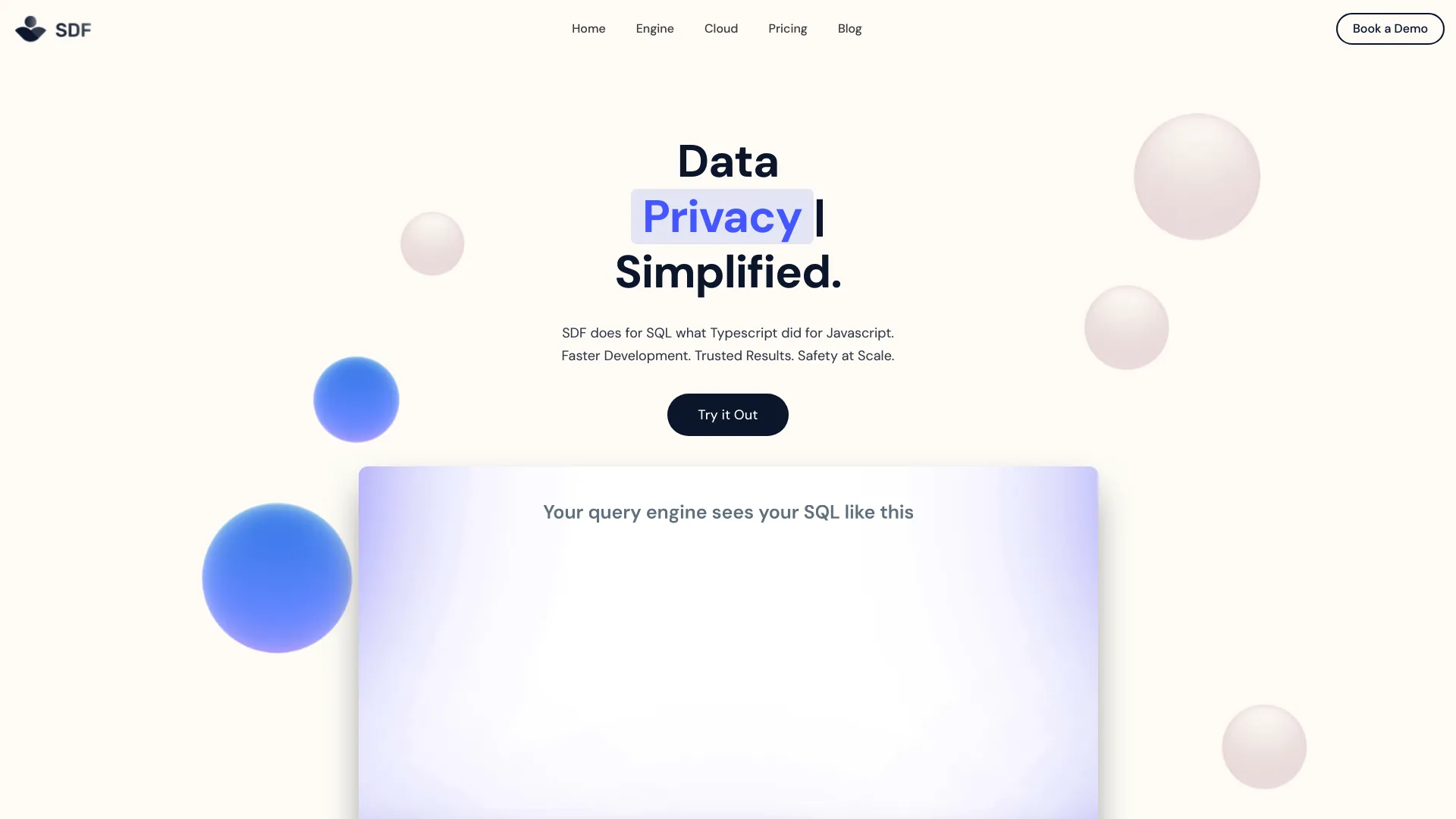
Introducing SDF: an innovative AI SQL development tool. Designed to simplify SQL development, SDF offers intelligent workflows, advanced column-level lineage analysis, and robust data governance features. With a compact size of only 60MB and built on the powerful Rust framework, it aims to dramatically enhance the speed and efficiency of SQL development. SDF functions as a scalable build system for data infrastructure, similar to Cargo for Rust or Node for Javascript. By seamlessly integrating data contracts, queries, and policies, SDF enables code-level, time-level, and access-level dependencies. Its integrated scheduler handles backfills effortlessly, while lightning-fast local execution, data workspaces, and crystal-clear error messages pave the way for intuitive authoring, resulting in a 10X faster development speed. Simplifying warehouse deployment with a single command, SDF ensures compliance through label propagation, robust data policies, and native enforcement. Moreover, it provides a comprehensive data catalog with precise column-level lineage analysis. Regardless of organizational scale, SDF empowers businesses to maximize the utilization of their data while adhering to relevant policies and protections.
Introducing SDF: The AI SQL development tool for 10X faster development speed.
No items found.
Unfortunately this listing has not yet been claimed. We strive to verify all listings on Dang.ai and this company has yet to claim their profile. Claiming is completely free and helps us ensure that all of the tools listed on Dang.ai are up to date and provide as much information to users as possible.
Is this your tool?
Unfortunately this listing has not yet been verified. We strive to verify all listings on Dang.ai and this company has yet to claim their profile. Verifying is completely free and helps us ensure that all of the tools listed on Dang.ai are up to date and provide as much information to users as possible.
Is this your tool?
8.4.2023
This tool is no longer approved.
Dang.ai attempted to contact this company to verify this companies information and the company denied our request to verify the accuracy of their listing.