AI Behavioral And Neural Data Analysis Tool
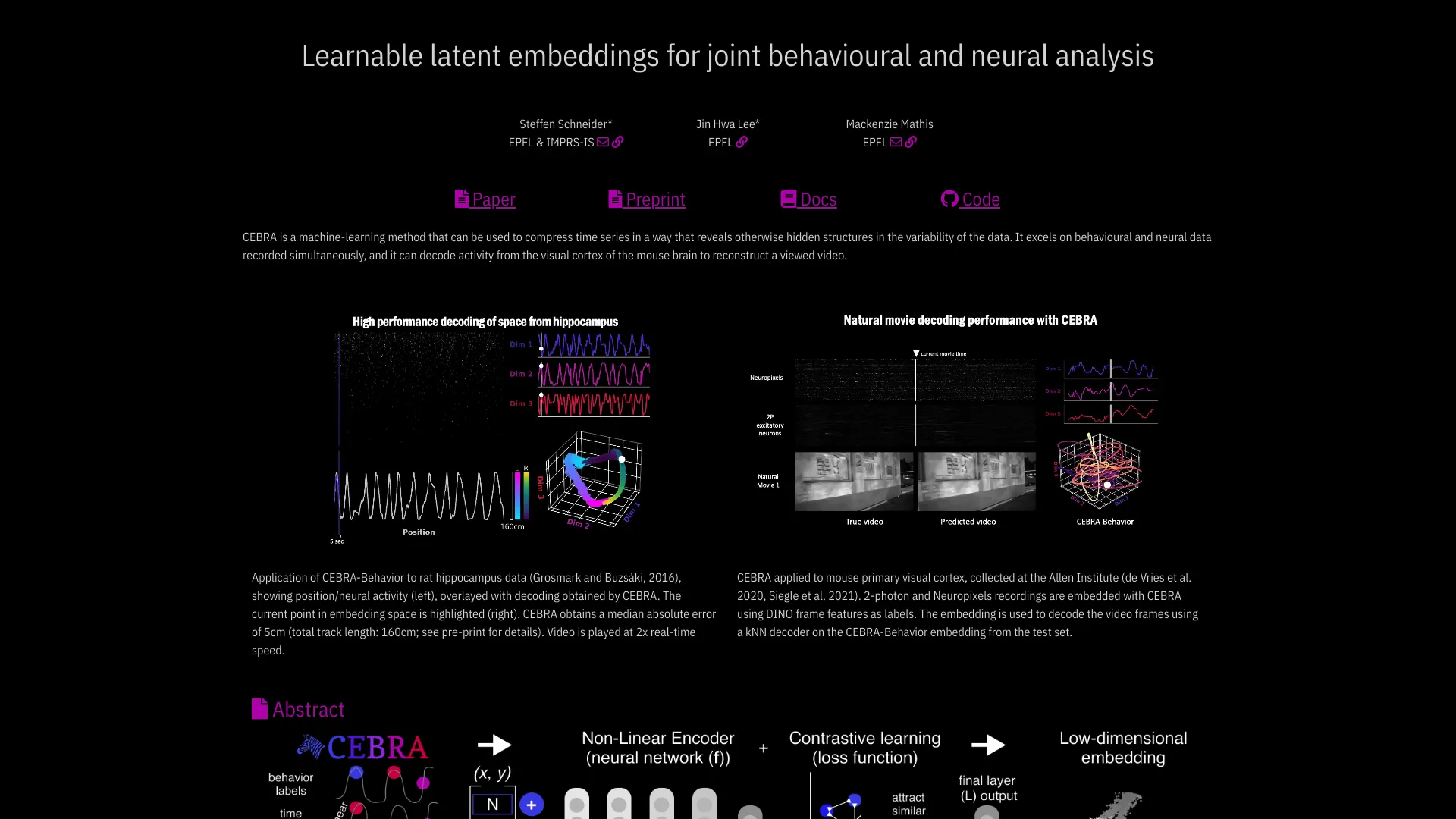
What is cebra.ai?
CEBRA is a machine-learning technique tailored to compress time series data, revealing latent structures within its variability. It excels in analyzing concurrent behavioral and neural data. Notably, CEBRA showcases a unique ability to decipher neural activity from the visual cortex of mice to reconstruct observed videos.
Its versatility extends to both supervised and self-supervised approaches, facilitating hypothesis testing or exploratory research. Extensively validated for precision, CEBRA proves beneficial across diverse datasets, tasks, and species. Additionally, it aids in spatial mapping, intricate kinematic feature identification, and swift, accurate decoding of natural visual stimuli from the cortex.
How does cebra.ai work?
CEBRA, or Compressed Encoding of Behavioral Representations in Arrays, utilizes machine learning techniques to compress and analyze time series data. Here's a simplified breakdown of its operation:
Data Collection: CEBRA gathers high-dimensional time series data, such as behavioral or neural data, from experiments.
Compression: The algorithm compresses this data, reducing its dimensionality while retaining essential temporal patterns and structures. This step enhances manageability and emphasizes significant features.
Machine Learning: Employing machine learning methods, CEBRA identifies patterns within the compressed data. It operates in both supervised (with labeled data) and self-supervised (without labeled data) modes.
Decoding: In cases involving neural data, CEBRA decodes the activity to reconstruct stimuli or predict behaviors. For instance, it can recreate a video by decoding the visual cortex activity of a mouse.
Analysis: Researchers analyze the decoded data to test hypotheses or uncover novel insights into brain function and behavior.
CEBRA's capacity to handle large datasets and unveil concealed structures makes it invaluable for neuroscience research and other domains dealing with intricate time series data. It proves particularly adept at elucidating variability in behavioral and neural recordings, potentially leading to breakthroughs in understanding how the brain processes information.
What are the benefits of cebra.ai?
CEBRA, a notable machine-learning method, offers numerous advantages for the analysis of behavioral and neural data:
Compressed Encoding of Data: CEBRA compresses time series data, unveiling concealed structures within its variability. It adeptly processes concurrent behavioral and neural data.
Joint Behavioral and Neural Analysis: CEBRA can decode activity from the visual cortex of the mouse brain to reconstruct viewed videos. For instance, it can recreate a video by decoding neural activity patterns.
Latent Embeddings: CEBRA generates latent embeddings, representing high-dimensional data in lower dimensions. These embeddings capture meaningful relationships and structures within the data.
Consistency and High Performance: CEBRA consistently produces high-performance latent spaces. It proves effective for calcium imaging and electrophysiology datasets, spanning sensory and motor tasks, and various behaviors across species.
Flexible Use Cases:
- Hypothesis Testing: Researchers can employ CEBRA for hypothesis testing using labeled data.
- Discovery-Driven Science: CEBRA facilitates self-supervised learning, revealing latent structures without predefined labels.
- Mapping Space: It can map spatial features.
- Kinematic Features: CEBRA uncovers complex kinematic features.
- Decoding Natural Movies: It swiftly and accurately decodes natural movies from the visual cortex.
In summary, CEBRA enables researchers to delve into neural dynamics, comprehend behavior, and unveil hidden patterns, rendering it a valuable asset in neuroscience and beyond.
What are the limitations of cebra.ai?
While CEBRA.ai offers powerful capabilities for time series data analysis, it also comes with certain limitations:
Data Requirements: CEBRA necessitates substantial amounts of data for effective training, posing challenges when data availability is limited or collection is arduous.
Potential for Bias: There exists a risk of bias in the analysis, a common concern in machine learning applications, which can impact the accuracy and fairness of results.
Complexity of Interpretation: The compressed encoding and latent embeddings produced by CEBRA may be intricate to interpret, requiring users to possess a solid understanding of machine learning and data analysis to extract meaningful insights.
Generalization: While CEBRA has demonstrated efficacy across diverse datasets and tasks, its ability to generalize to all types of behavioral and neural data is not guaranteed. Performance may vary based on the specific characteristics of the data.
Computational Resources: Processing and analyzing large datasets with CEBRA demand significant computational resources, which may not be readily accessible to all researchers.
These limitations should be carefully considered when evaluating CEBRA's suitability for a particular research project. Despite these challenges, CEBRA remains a valuable method for uncovering hidden structures within complex time series data.
How do I get started with cebra.ai?
Starting with CEBRA.ai is a simple process. Here's a step-by-step guide to help you get started:
Learn About CEBRA: Begin by understanding what CEBRA is and how it functions. Visit the official website and explore relevant literature, such as the preprint paper on arXiv.
Access the Code: The official implementation of the CEBRA algorithm is accessible on GitHub. You can watch and star the repository to receive notifications about future updates and releases.
Set Up Your Environment: Ensure your computational environment is configured correctly. This typically involves installing Python and necessary libraries and dependencies.
Download the Dataset: If you have your dataset, you can utilize it. Alternatively, access public datasets compatible with CEBRA for practice purposes.
Run CEBRA: Follow the instructions provided in the GitHub repository to execute CEBRA on your dataset. The repository includes examples and documentation to guide you through the process.
Analyze the Results: Upon running CEBRA, analyze the generated output to comprehend the latent embeddings and their implications for your data.
Stay Updated: Keep abreast of developments in the CEBRA project by following them on social media or subscribing to their mailing list. For potential collaborations, consider contacting the team via email.
Remember, if you encounter any challenges or have inquiries, the CEBRA community, including developers, can offer valuable assistance. Feel free to seek support or clarification as you explore this potent data analysis tool.